A team comprised of lecturer Keiko Imamura, guest researcher Yuichiro Yada, and Professor Haruhisa Inoue of the Center for iPS Cell Research and Application, Kyoto University (CiRA) have developed an AI model capable of differentiating iPS derived motor neurons of both healthy individuals and patients with ALS (amyotrophic lateral sclerosis) with a diagnostic precision of 97%. Inoue explains, "Deep learning carries out feature extraction automatically, so typically the judgement criteria being used is a black box, and cannot be applied to unknown data. Moving forward, we hope to add even more data to use this as a system to support diagnosing ALS in the future." The results were published in the Annals of Neurology.
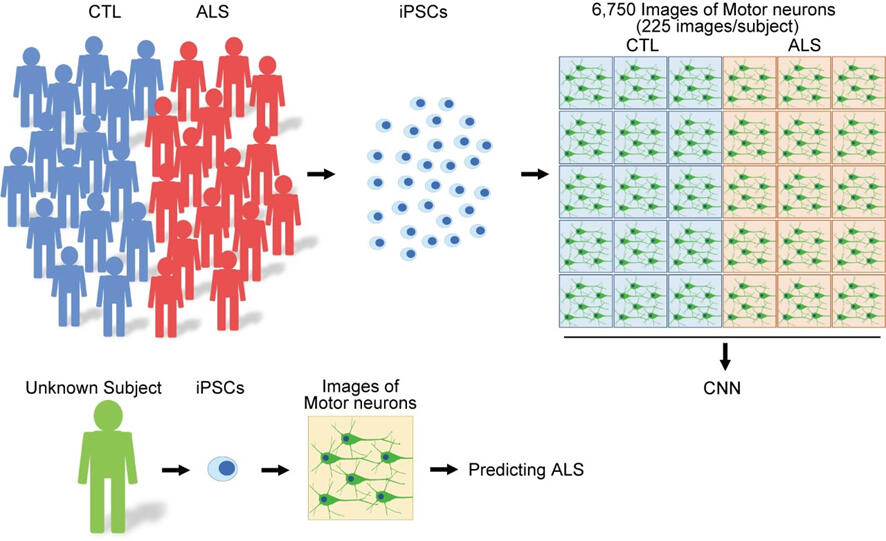
ALS is a disease that occurs in middle age and later in which motor neuron death causes progressive muscle weakness and atrophy, ultimately leading to death from respiratory muscle paralysis within two to five years after the onset of the disease. There are approximately 9,000 ALS patients in Japan. No fundamental treatment or preventive methods have yet been established.
ALS is a disease in which the symptoms progress rapidly after onset, but it typically takes 13.1 months on average in Japan from the onset to diagnosis of ALS by a medical doctor.
The research group gathered a total of 6,750 photographs of iPS derived motor neurons from both 15 healthy individuals and ALS patients, and used the data to teach a deep learning algorithm. They then fed the learning results into an AI model, and found a diagnostic precision of 97.4% when it was used to examine the iPS derived motor neurons of other ALS patients.
Then the team used the Gradient-weighted Class Activation Mapping (Grad-CAM) method to determine what part of the motor neurons the AI model was focusing on for its diagnoses, and found that the answer was the cell body and neurites. They also found a relationship between diagnostic precision and disease duration for the given patient, such that the AI tended to have an easier time diagnosing the motor neurons the longer the patient had been suffering from the disease. In comparison, diagnostic precision stopped at around 60% when traditional factors, such as neurite length, the area of nerve cell body, and the number of neurons, were analyzed using machine learning (specifically the Random Forest method).
Inoue adds, "Under the current model, it takes three weeks to produce the iPS cells and then derive the motor neurons, but if the model can be used to support diagnosis, it would make it possible to produce a diagnosis much more quickly. While only symptomatic treatment is possible right now, earlier diagnosis might lead to the ability to slow down disease progression, or even accelerate healing in the future."
This article has been translated by JST with permission from The Science News Ltd.(https://sci-news.co.jp/). Unauthorized reproduction of the article and photographs is prohibited.