NTT has developed a new deep learning technology that can learn unknown three-dimensional (3D) information (depth and bokeh blur effect) from general groups of photographs. Based on their experiences and knowledge, humans can relatively easily estimate 3D information from corresponding two-dimensional (2D) images such as photographs. However, as computers have no such experience or knowledge, it has not been easy to develop understanding of 3D information from only a 2D image until now. However, a deep layer learning technology that enables this understanding has just been developed for the first time in the world. This is a milestone in advancing our understanding of the 3D computational world. From a practical perspective, it is expected to significantly reduce the cost of collecting data. The result has made it possible to learn 3D information, such as depth and blur effects, only from general groups of photographs, such as images published on the Internet, by newly constructing a deep learning model considering the optical constraints in the images captured by a camera.
In this study, researchers aimed to create a computer that can understand 3D information from only 2D images, which are relatively easy to obtain. The focus was on the bokeh blur effect, which naturally exists in images taken with a conventional camera, after considering the type of clue to provide to a computer to allow it to understand 3D information.
This blurring effect is a phenomenon caused by the subject deviating from the depth of field (the range of the distance on the subject side where the photograph appears to be in focus), and the degree of blurring can be changed by adjusting the aperture of the camera. Specifically, when the aperture is wide, the depth of field becomes shallow (the area in focus becomes narrower) because the amount of light entering the camera increases. Thus, the blurring effect (the effect of the depth of field) increases. Conversely, when the aperture is narrowed, the amount of light entering the camera reduces. Hence, the depth of field becomes deeper (the area in focus becomes wider), and the blurring effect decreases. Thus, the aperture of the camera plays an important role in reflecting the 3D positional relationship of the subject (specifically, the distance from the camera, i.e., the depth) and the image as a blurring effect.
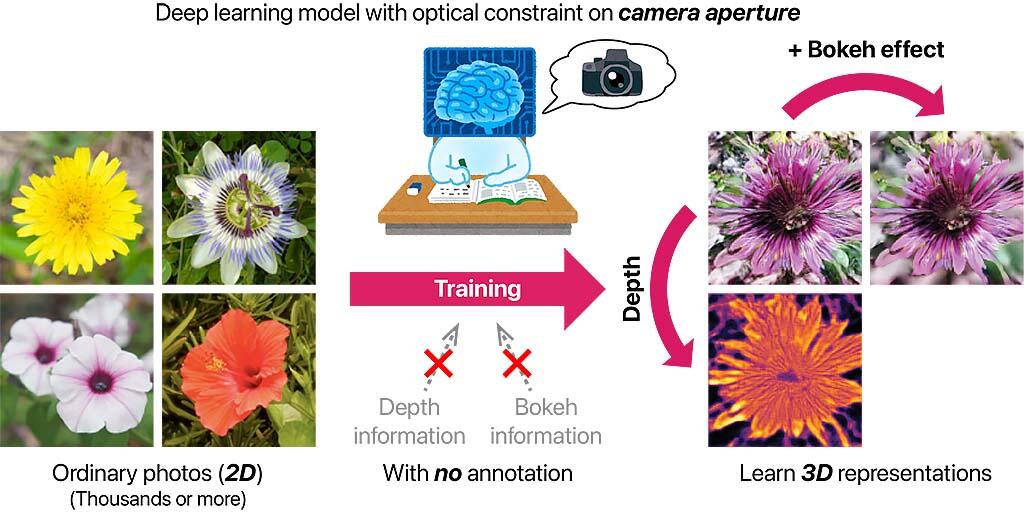
Credit: NTT
Based on this idea, researchers in this study constructed a new deep learning model that considers the optical constraints on the aperture of the camera. They enabled the model to learn while associating the three viewpoints of an image, depth information, and the bokeh effect. Consequently, even though only general photographs without additional information were used during learning, the model reproduces the photographs during the process of learning, enabling it to be trained on information about the depth structure of a scene and the bokeh effect included in photographs. This is the first study to report a model that can successfully learn 3D information, such as depth and the blur effect, from only 2D image sets without requiring any additional information. When using conventional technology, 3D information needs to be collected using a dedicated device, meaning that the data collection cost is high. In contrast, the advantage of this technology is that such information can be learned only from general photographs, such as public images on the Internet.
This article has been translated by JST with permission from The Science News Ltd.(https://sci-news.co.jp/). Unauthorized reproduction of the article and photographs is prohibited.