A research group led by Professor Akimasa Hirata of Nagoya Institute of Technology has trained a model to predict the spread and convergence of novel coronavirus infections, particularly the number of newly infected persons, by using movement data and meteorological data from several prefectures between March 2020 and June 2021. They applied a recurrent neural network called LSTM, which is a deep learning-based artificial intelligence technology that is useful for handling time series data. The rate of decrease in the movement of people and the time series data of the weather were input, and the number of newly infected persons (weekly average value) for each day over the following month was learned and applied for the prediction. By standardizing the data for Tokyo, Aichi, Osaka, Hyogo, Kyoto, and Fukuoka prefectures, the model was able to predict outcomes with an accuracy of approximately 80% as long as the effects of mutant strains remained small.
Data after the emergence of the alpha variant also showed that approximately 4 weeks of data accumulation was required to provide sufficient prediction accuracy for the novel variant. In these cases, the estimated effective reproduction rate and the estimated target rate of the increase or decrease in human flow can also be calculated based on the statistics of the increase or decrease in human flow in the past week, including the incubation period.
Data up to June 30 showed that under the same population movement patterns, the effective reproduction numbers of the alpha and delta strains were 20-30% and 20-40% greater, respectively, compared to those of the conventional strain. Mathematical models (SEIR) are generally used to predict the number of newly infected people, but the predicted values may deviate significantly because differences in regions, cultures, environments, and other related factors, are not taken into consideration. Moreover, Professor Hirata's model showed good agreement when the predicted values and actual numbers for each region were compared.
Professor Hirata said, "The SEIR model is suitable for deriving rough trends, but LSTMs are more accurate when it comes to detailed forecasts. When recommending behavior change to combat infectious diseases, I think we need to make good use of both models to convince many people."
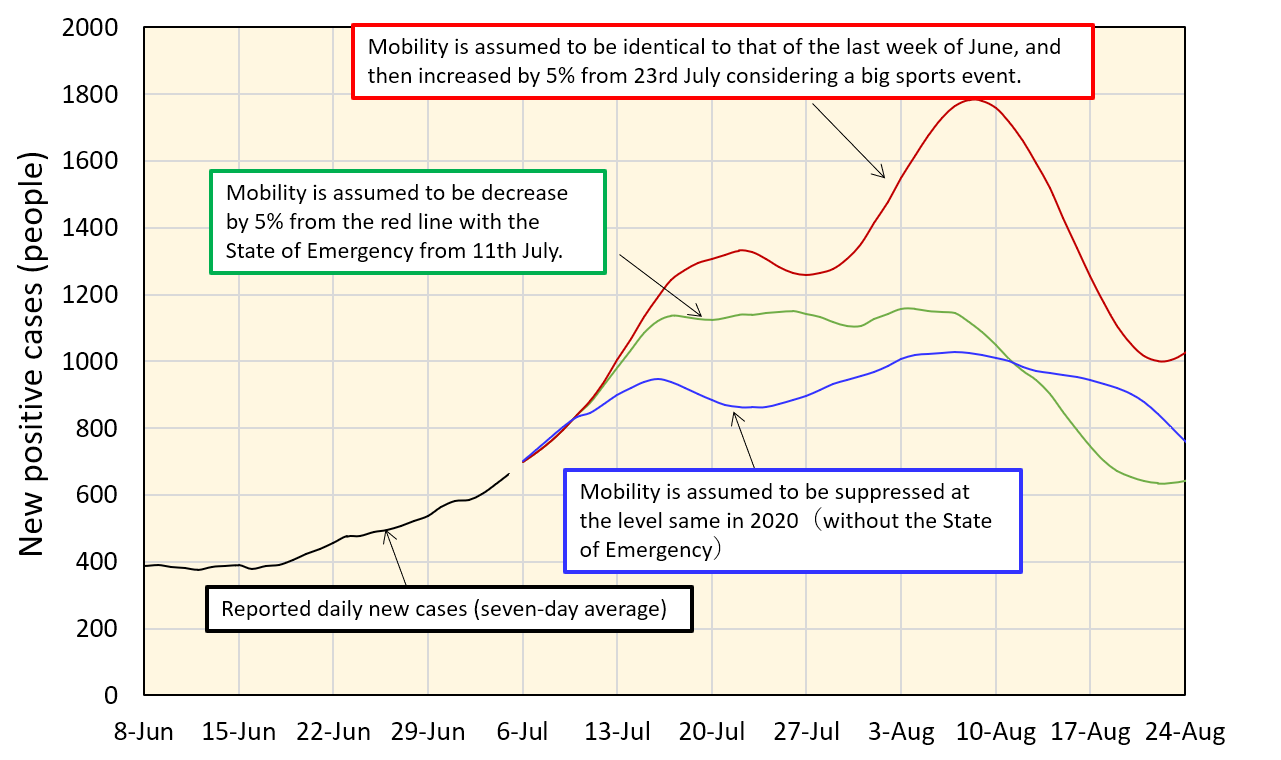
Reported and estimated values indicate a 7-day average centered on the day.
Credit: Nagoya Institute of Technology
This article has been translated by JST with permission from The Science News Ltd.(https://sci-news.co.jp/). Unauthorized reproduction of the article and photographs is prohibited.