Chief Scientist of the High Energy Nuclear Physics Laboratory at the RIKEN Cluster for Pioneering Research, Takehiko Saito, in collaboration with Gifu University, Tohoku University, Rikkyo University, and Instituto de Estructura de la Materia (IEM, Spain), announced the successful visual detection of the formation and collapse events of a "hypertriton," which is a type of hypernuclear system. This was achieved by analyzing photographic plate data irradiated with a kaon beam in the Japan Proton Accelerator Research Complex (J-PARC) using a machine learning model that was developed for the research project. This achievement is expected to lead to the clarification of the origins of substances. The group's results were published in the September 14 issue of Nature Reviews Physics.
The nuclei that make up an atom are formed by binding positively charged protons and uncharged neutrons with a nuclear force. However, the interactions (nuclear force) between neutron and proton particles are not well understood, and research on a special atomic nucleus called the "hypernucleus" is being conducted to clarify these mechanisms.
A hypernucleus contains particles called "hyperons" in addition to the protons and neutrons that make up ordinary nuclei. The nuclear force is studied by investigating how these differences affect the properties of nuclei. The lightest hypernuclei are called "hypertritons" and are formed of a proton, a neutron and a lambda (Λ) particle. Yet, the mystery of the "hypertriton puzzle" remains unexplained.
This is due to the experimental result that the lifetime of "hypertritons" from generation to collapse is significantly shorter than the value predicted from the binding energies measured in the 1970s. Since this experiment, several other experiments have been conducted at various research institutes around the world, but the measured values have varied, and no conclusive results have been found. In addition, the binding energy of the hypertriton used in this experiment is based on the measured value obtained using "photographic emulsion techniques" in 70's. However, in 2020, results using the electron-ion collider during the STAR experiment at Brookhaven National Laboratory in the United States indicated that the actual values could be several times larger than the values measured 50 years ago.
In this research, the group used the data from emulsion photography (modern dry plates) irradiated with a kaon beam (1300 shots) obtained in the E07 experiment conducted at J-PARC from 2016 to 2017, which generated hypertritons and searched for collapse events. This special photographic plate that can record traces of charged particles as tracks is known as nuclear emulsion. It can be used to measure the 3D position information with an accuracy of 1 μm or less and the binding energy for each event with a high accuracy based on observations using an optical microscope. By contrast, as a dry plate records the tracks of all particles that passed it, from immediately after manufacture to development (10 million images per dry plate), it contains a large amount of information in addition to the information to be measured. Even with a program that searches for candidate trajectories, the amount of information collected would have required 560 years of analysis.
To counter this issue, the research group considered using machine learning to detect objects in an image. Using an object detection method called the mask R-CNN, the research group developed a machine learning model for detecting traces of the collapse of a hypertriton. As hypertriton events were extremely rare in the first place and the required amount of data did not exist, the research group considered creating new data using physics simulations (Monte Carlo simulations) and image conversion technology to use as training data. Specifically, after the hypertriton hits the photographic plate, it loses kinetic energy and stands still, then then they decay into two particles - a pion and a helium-3 nucleus - which is simulated and the track information is output.
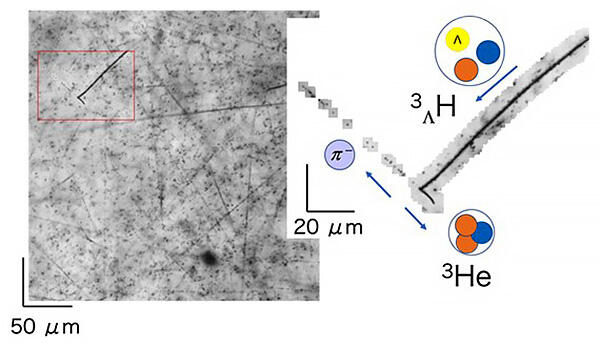
Credit: RIKEN
In order to convert this data into a simulated image, similar to a microscope image of a photographic plate, a generative adversarial network (GAN) was used. The simulated images obtained using the GAN were used as training data to train mask R-CNN, and the actual photographic plate images were then analyzed.
As a result, the research group succeeded in discovering a hypertriton event for the first time on February 2, 2021. The event causes K-minus particles to come to a halt and be absorbed into the nucleus, releasing other particles, including a hypertriton. The hypertriton then flies approximately 0.7 mm and stops, releasing pions (π) and helium-3 nuclei. The π-minus particle travelled approximately 29 mm and stopped, and the helium-3 nucleus travalled approximately 8 μm and then stopped. The kinetic energy calculated from the trajectory length and the kinematic analysis confirmed that this was a hypertriton.
To date, 14 hypertriton events have been discovered. Once approximately 50 cases are found, it is expected that the binding energy can be measured. Going into the future, the research group plans to measure the lifetime of the hypertriton with the highest accuracy to date at GSI in Germany in February 2022. If this reveals the lifetime of hypertriton, it is expected to solve the "hypertriton puzzle" and clarify the nature of the fundamental interactions between particles.
This article has been translated by JST with permission from The Science News Ltd.(https://sci-news.co.jp/). Unauthorized reproduction of the article and photographs is prohibited.