One in ten Japanese office workers suffer from back pain. This lower back pain (LBP) may be aggravated by sitting for a long time, even if a good posture is maintained. If the occurrence of this pain can be predicted, actions such as stretching and other exercises can be used to alleviate the issue.
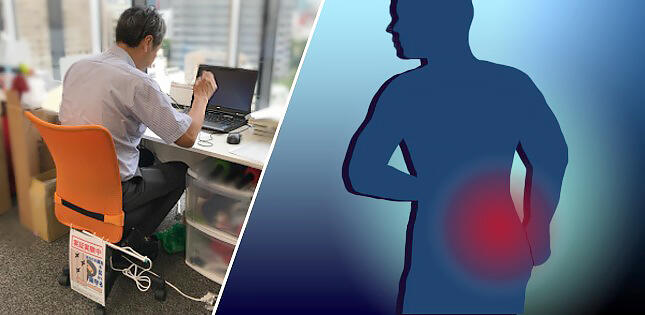
Professor Ryoichi Nagatomi, Specially Appointed Lecturer Keizo Sato and Wang Ziheng, and other graduate students of the Graduate School of Biomedical Engineering, Tohoku University, used a "smart chair" to discover a common pattern of fine movements in the trunk that may prevent fixation of vertebral joints by analyzing the changes in the weight-bearing signals while sitting, with the help of artificial intelligence (AI); they found that LBP worsened with a high probability when this pattern was absent. These findings were published in the online version of Frontiers in Physiology.
The research group collected data over three months from 22 office workers who consented to participate in the study and had their subjective degree of lower back pain recorded on a tablet terminal three times a day. From the data of load fluctuations, while sitting, they identified signal changes common to 22 individuals utilizing layers of deep learning and further detected a combined pattern strongly associated with predictable LBP progession. Both sensitivity and specificity achieved a high predictive accuracy of nearly 70%, and results were obtained that can have clinical implications.
That is, the prediction of subjective lower back pain among sedentary office workers has been made possible by using AI analytical techniques, including deep learning from time-series signals corresponding to fluctuations of the center of pressure obtained from four weight-sensors installed under the seat of the office chair. Until now, posture and sitting time in people with a risk of chronic back pain owing to sitting in a chair have been researched using sensors. However, there was no technology for predicting subjective back pain from fluctuations in the center of pressure by sitting for several minutes. This was attributed to the difficulty in mathematical modeling of highly irregular time-series signals in real life.
However, applying the latest time-series signal processing and AI technology has made it possible to predict events such as lower back pain, which becomes a problem from the bio-derived stochastic signal system. This suggests the possibility of applications to task event prediction based on various forms of psychogenesis and perception, such as pain and discomfort. It was also shown that individualized prediction is possible by detecting patterns of time-series signals in individuals, unlike the previous risk factors in the form of indicators defined by range and degree of immobility.
In the future, the value of using sensors in wearables in real life scenarios may significantly increase. Moreover, by discovering fine movements that prevent joint fixation, the causes of symptoms known as "general mailaise" such as shoulder stiffness, headache, and joint pain may be clarified and methods of management further developed.
This article has been translated by JST with permission from The Science News Ltd.(https://sci-news.co.jp/). Unauthorized reproduction of the article and photographs is prohibited.