To develop materials with particular properties, the structure required for the material can be derived using material informatics (MI). When predication accuracy is high this process proceeds smoothly, however, actual development of the materials is not so simple. A research group led by Ryo Tamura, Senior Researcher, Takashi Nakanishi, Group Leader, and Masahiko Demura, Director, Research and Services Division of Materials Data and Integrated System, National Institute for Materials Science (NIMS); Yuki Takei, Senior Researcher, Asahi Kasei; Shinichiro Imai, Research Associate, Mitsubishi Chemical; Maki Nakahara, Researcher, Mitsui Chemical; and Satoshi Shibata, Researcher, Sumitomo Chemical, have effectively used the measured data obtained from the structure of materials and developed an AI technology that reduces the error between the predicted values and the actual values with a small number of experiments. This is expected to become a powerful tool for material development. The groups results were posted online in Science and Technology of Advanced Materials: Methods.
MI research has accelerated material development by predicting material physical properties via machine learning using the composition of materials and processes used in their formation (temperature, pressure, etc.). When the structure of a material affects its properties, it was effective to use measurement data such as X-ray diffraction (XRD) and differential scanning calorimetry (DSC), which provide structural information, to achieve high prediction accuracy. However, this kind of data could not be obtained unless processed materials were measured.
That is, to improve the prediction accuracy using structural information, it was necessary to deal with two parameters, the composition of the material, which can be controlled by the researcher, and those than can only be obtained through actual measurement.
First, using the AI technology developed in this study, one material was created from the candidate data composed only of descriptors that could be controlled by researchers, and measurement data such as XRD and DSC and the physical properties of the material were obtained. Second, as the initial data, a dataset including all the material properties and measurement data such as XRD and DSC of the same material as the candidate material was prepared, and a machine learning model for predicting material properties from measurement data was constructed. AI appropriately selected the candidate materials that were expected to increase the prediction accuracy of this machine learning, and the researchers manufactured the materials. Two selection methods were proposed by this AI: a method based on Bayesian optimization (BOED) and a method based on uncertainty sampling (USED).
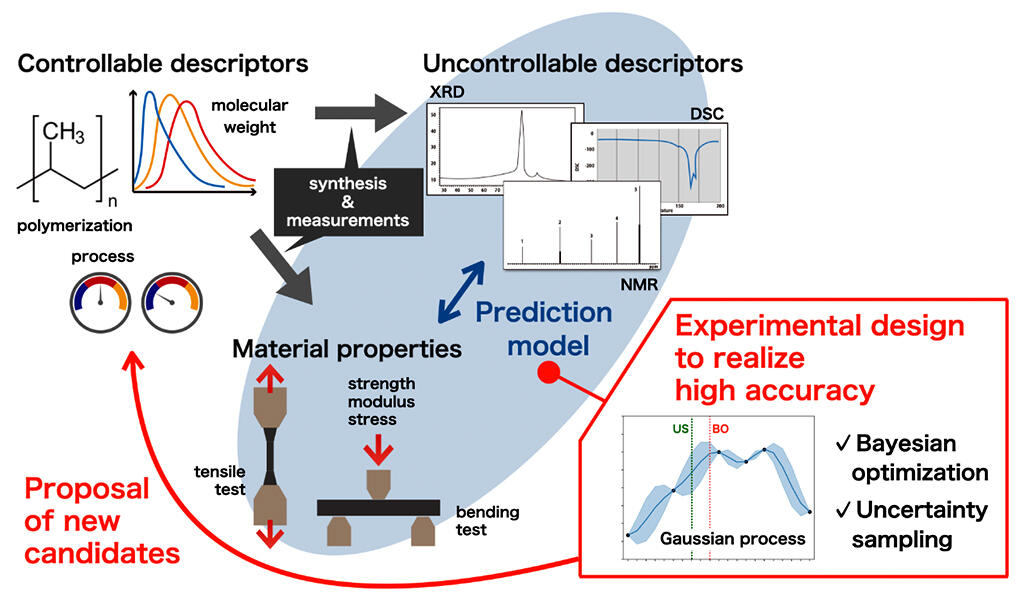
(Provided by NIMS)
To verify its effectiveness, a database created by chemical MOP (open platform of the four companies and NIMS) was used with polyolefin as the target material. The database consisted of 75 samples, obtained from 15 polypropylenes by five different processes. When the experiment was conducted taking the mechanical properties of the Charpy impact test and tensile modulus as prediction targets, compared to when the materials were made at random, the prediction error was reduced with a small number of material fabrications for all mechanical properties when the two AI techniques were used. A reduction in the number of material fabrications by improving prediction accuracy accelerated the material development.
Chief Researcher Tamura said, "The need for experimental design is rising in various fields, and we would like to develop a method that works regardless of the field. We believe that this method can be widely applied not only to polymer materials but also to material development in general."
The importance of AI continues to increase. In the future, more situations will arise where AI will be used for research and development. Just as measured data such as XRD and DSC played an especially large role in this research, the importance of accurate analysis and measurement technology is increasing in the use information technology. Collaboration between academia and industry, and between companies, is also a crucial factor in creating innovation.
This article has been translated by JST with permission from The Science News Ltd.(https://sci-news.co.jp/). Unauthorized reproduction of the article and photographs is prohibited.