AIST has developed the world's first method for building an image recognition model (learned model) for AI using an extensive image data set automatically generated from mathematical formulas. This method resulted from NEDO's (New Energy and Industrial Technology Development Organization) "Technology Development Project on Next-Generation Artificial Intelligence Evolving Together With Humans." AIST presented details at the IEEE/CVF International Conference on Computer Vision and Pattern Recognition (CVPR) 2022, in New Orleans.
This method not only achieves the same or better image recognition accuracy than current methods that use real images with training labels added by humans (images that have been tagged for training), but also solves challenges related to commercial AI recognition of image data, such as collecting large amounts of real image data for use in training and ensuring its privacy, as well as reducing the costs associated with human labeling. AIST expects that this method will be used for producing AI for various environments, such as automated driving, medical care, and logistics.
In some fields, such as manufacturing and medicine, it is impossible to collect the large amount of data necessary for AI to learn, or high costs are required to do so, creating barriers to introducing AI technologies. One method of overcoming these barriers is utilizing image recognition models that use AI pre-trained with large amounts of varied real image data (learned models). However, there are issues related to data transparency, such as privacy violations depending on the images used for training and improperly assigned training labels that output unfair recognition results. These issues have become an issue for commercial use of these AI systems.
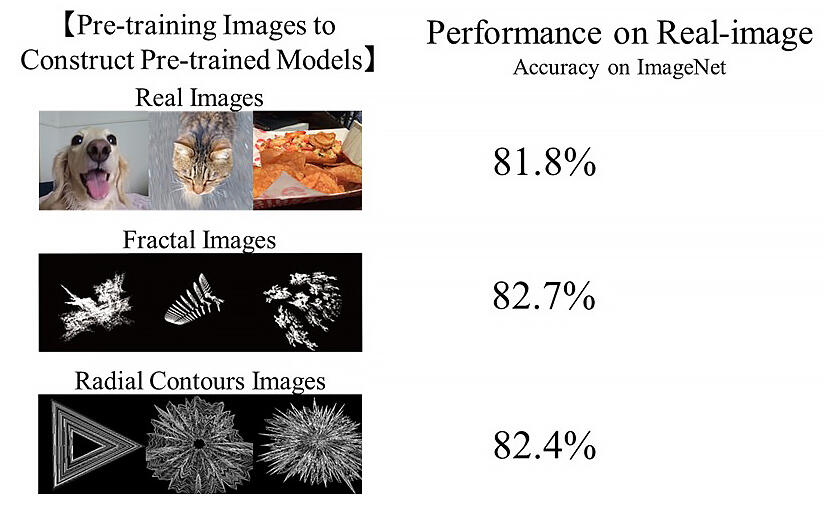
Provided by AIST
Therefore, developing trained models with recognition accuracy that is at least as good as conventional models while solving these aforementioned image data-related problems has become an urgent issue in the field of AI. AIST's methods reduce labeling costs by automatically generating image patterns and training labels from mathematical formulas (generation rules for generating image patterns and training labels) without using any real images in the preliminary training and worrying about the numbers of real images, ethical issues, or rights issues.
AIST has also confirmed that the trained models constructed with this new method are superior to current methods that use real images and human-judged training labels in recognizing ImageNet image datasets (a benchmark for image recognition performance) and can be used for real-world use. The dataset and trained models were made available on the group's website (Formula-driven Supervised Learning) on June 13. By releasing trained models built using the new method, users can start development with AI that already understands imagery to the required level of accuracy.
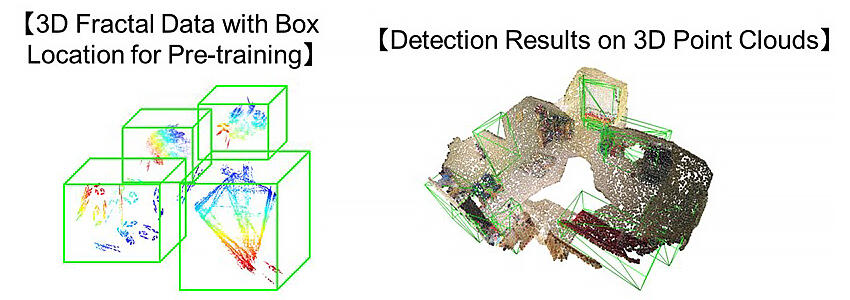
Provided by AIST
This article has been translated by JST with permission from The Science News Ltd.(https://sci-news.co.jp/). Unauthorized reproduction of the article and photographs is prohibited.