Fusing chemistry and AI requires creating a database that is industry accessible and revising the educational curriculum from the undergraduate level. Associate Professor Miho Hatanaka from Keio University's Department of Chemistry, Faculty of Science and Technology, proposed this idea at the Council for Science, Technology and Innovation's Expert Panel Meeting. Many councilors responded favorably to the concept, which will be incorporated into future policies promoting advanced research.
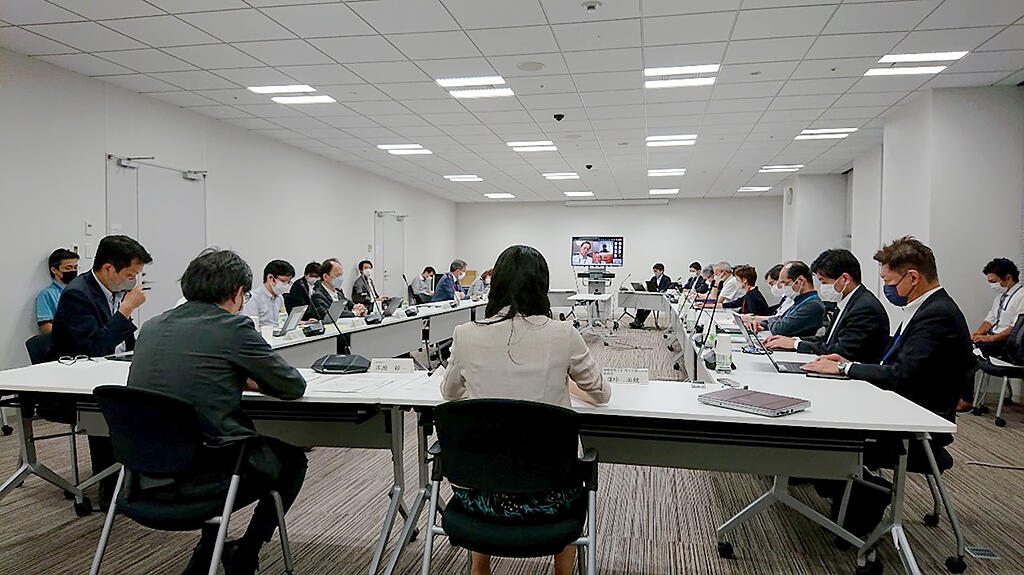
After starting her career in experimental chemistry, Associate Professor Hatanaka has worked in theoretical and computational chemistry, simulations, and the use of AI and quantum computers in chemistry. Some of her achievements include developing energy-shifting methods and utilizing machine learning to significantly reduce the design cost of complex optical functional materials, along with theoretical research into chemical reactions and catalytic reactions, and she has received high international acclaim.
Advances in theoretical and computational chemistry and computing technology have made it possible to design mechanism-based materials. However, complex catalytic reactions, rare metal materials, and biomolecule mimetic materials are either impossible to calculate or, even if they could be calculated, would require decades to complete. These limitations have led to stagnation in this field. Against this backdrop, Professor Satoshi Maeda of Hokkaido University (currently Director of the Institute for Chemical Reaction Design and Discovery (ICReDD) has helped re-energize this field by developing a theoretical technique to systematically and automatically search for unknown elementary reaction processes using quantum chemical calculations. Automated reaction pathway search technologies have made it possible to automate the visualization of complex chemical reaction processes. As a result, Japan is now leading the way in technologies to simulate chemical reactions. However, these advancements do not solve the problem of materials that cannot be calculated or require excessively long calculation times.
This is where AI (deep learning) predictions have been gaining attention in recent years. Large amounts of input and output data would allow for predictive systems to be built. For example, vast material and process data sets would enable the creation of an AI-based material performance prediction system. Collecting data sets possessed by Japanese companies would enable this system to be built, but since this data is confidential to each company, it cannot be shared throughout Japan.
To address this issue, Hatanaka proposes constructing a shared-use database. For example, when designing a process for a chemical product that uses a catalyst, a database of 3,000 to 4,000 catalytic reactions could be automatically searched for reaction pathways. This process could then be used by the company. Companies would build AIs with catalytic reactions in the database as input and in-house experimental data as output. By keeping these databases private, each company could discover the most appropriate catalysts on its own.
However, human resources are essential to advance development and fuse AI and chemistry at each company. Hatanaka said, "Collaborative research cannot proceed simply by understanding your field and the other party understanding their field. Progress can be made only when you have some expertise in the other party's field and can discuss things from the same perspective and at the same level as them. I first studied materials chemistry, followed by theories for simulation, then simulations and AI. However, in the future, it will be essential to develop the type of human resources with multiple areas of expertise--understanding of materials chemistry and AI--and can provide overall coordination. Even without that level of understanding, we need to understand what experimental data can be used in AI."
Hatanaka independently teaches programming, machine learning, and other subjects to her lab's students but says there are limits to what she can do alone. Keio University is promoting an extra-curricular initiative that allows first-year students from other faculties and departments to attend lectures on these topics. Hatanaka added, "Many more universities need to introduce on-demand delivery of lectures from other departments and faculties, as well as revamp their curriculums to provide a double major system." She also said that a solid understanding of mathematics at the high school level is essential. "Physics requires an understanding of mathematics. For this reason, gaining a proper understanding of mathematics at high school is vital."
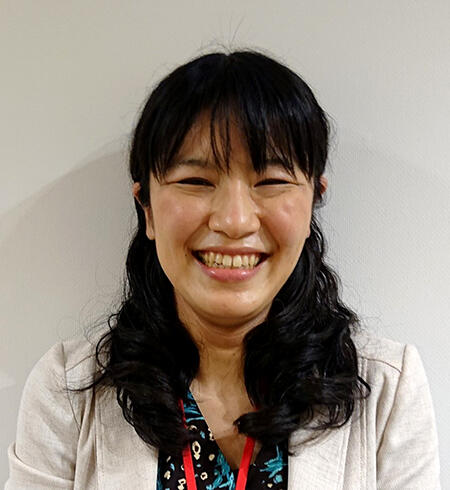
This article has been translated by JST with permission from The Science News Ltd.(https://sci-news.co.jp/). Unauthorized reproduction of the article and photographs is prohibited.