A research group has shown that introducing variation in neuron characteristics into a reservoir computing model--a type of artificial neural network--can improve time series prediction performance in multiscale systems. The group's members included Project Associate Professor Gouhei Tanaka, and Professor Kazuyuki Aihara from the University of Tokyo's International Research Center for Neurointelligence (WPI-IRCN), along with researcher Tadayoshi Matsumori and senior researcher Hiroaki Yoshida who work in mathematical engineering at Toyota Central R&D Labs. Their development is expected to become another time series forecasting technology for the various multiscale systems that exist in the world, such as the brain/nervous system and atmospheric/oceanic systems. The findings were published in the journal Physical Review Research.
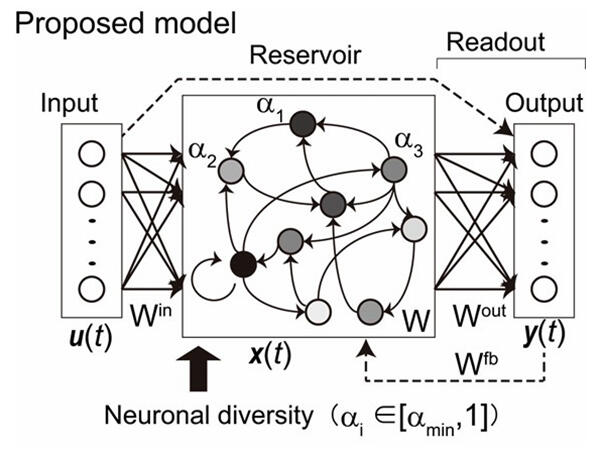
Provided by WPI-IRCN
In the real world, there are many multiscale systems in which multiple subsystems with different temporal and spatial scales reciprocally interact. For example, cells and organs in biological systems, the atmosphere and oceans in meteorological systems, and molecules and crystals in material systems affect each other while showing state variations on different spatio-temporal scales. The time series data observed from these multiscale systems include multiple, differing time scales. This characteristic limits analysis through existing methods for machine learning-based time-series predictions. Even the reservoir computing method, which has recently been attracting attention for its fast and easy learning, has difficulty representing diverse multiscale dynamics in a single model because the range of time scales is limited in conventional models that use the same group of neurons.
In the present study, the group intentionally applied characteristic variations to a group of neurons in the reservoir computing model to achieve a machine learning model that can generate variation over various time scales. In other words, the group used a heterogeneous group of neurons to add diversity to the reservoir's components.
Model analysis revealed that increasing the neuron population heterogeneity increased the model's time-scale distribution range. Next, the group artificially generated time series data from four multiscale systems consisting of fast and slow subsystems and performed a forecasting task using the proposed model.
Numerical experiments showed that the proposed model could improve prediction accuracy compared to conventional models in the single-step-ahead prediction task, in which the whole system is predicted using only fast subsystems. In the long-term forecasting task, the proposed model could forecast more extended periods than the conventional model. In other words, this is an example of how variation in the characteristics of neurons can help improve the time series prediction performance of artificial neural networks.
In the researchers' proposed reservoir computing model, diversity is introduced into the group of neurons by stochastically distributing a parameter, called leak rate, which each neuron possesses. In this study, a log-normal distribution was assumed to simplify the method, but any other probability distribution can be applied, and models with various time scale distributions can be realized.
The research group will further verify the effectiveness of the proposed method using actual data from multiscale systems such as cranial nerve signals and climate change prediction. In addition, Toyota Central R&D Labs and the University of Tokyo's Social Cooperation Research Departments will study applying the technique to analyzing climate change.
This article has been translated by JST with permission from The Science News Ltd.(https://sci-news.co.jp/). Unauthorized reproduction of the article and photographs is prohibited.