The amount of information in researchers' laboratory notebooks, which are used to record research results, is enormous. Analyzing this data is both time-consuming and labor-intensive. At the same time, academic papers, patents, and other publicly available literature often omit conditions such as temperature and humidity at the time of the experiment, when reagents were synthesized, and the results of failed experiments, so experiment results are not comprehensive. Due to this, researchers have long desired a system enabling integrated management and sharing of all information related to scientific research.
A research group led by Dr. Kan Hatakeyama has developed an artificial intelligence-based system that records the daily experimental results of chemical and material science experiments as electronic laboratory notebooks and used automated data analysis on them. The system records relationships between information as graphs with connected dots and lines. This data format is similar to the concept of flowcharts, which researchers are familiar with, and it is easy for AI to process, making it ideal for both the AI and researchers. For analysis of graphical data, fingerprint algorithms for determining the presence or absence of specific experimental manipulations are employed to quantify the database in a way that is easy for humans to understand.
Dr. Hatakeyama's group also performed experimental research on solid polymer electrolytes using the newly developed system and evaluated its effectiveness. As a result, the group was able to clarify the optimal manufacturing process for solid polymer electrolytes, which exhibit conductivity close to that of liquids at room temperature, as well as the fundamental mechanisms behind their high performance. The future focus will be on the system's use in various materials research and its contributions to the digitization of research and open science.
Digital laboratory notebook and data analysis scheme
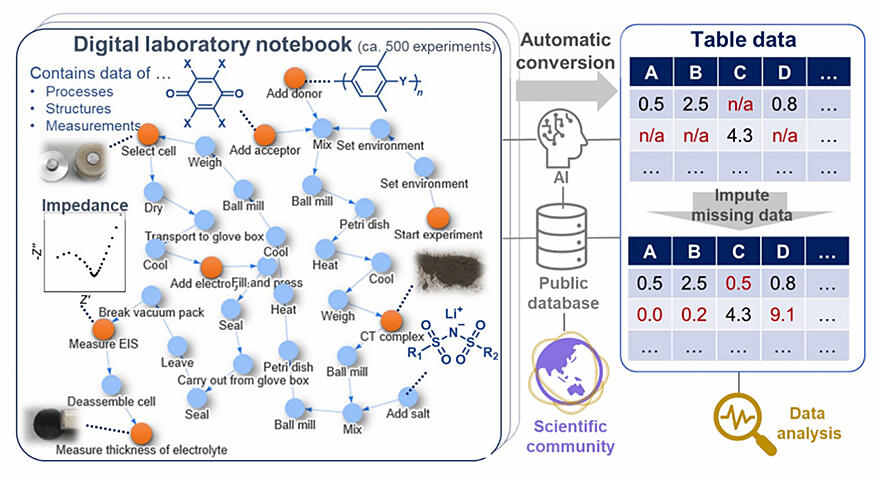