A joint research group led by Professor Keiichi Goto of the Department of Fisheries, School of Marine Science and Technology, Tokai University, and Fujitsu Ltd., has succeeded in a world first by non-destructively evaluating the freshness of frozen tuna, an essential quality indicator, using ultrasound AI technology.
Demand for high-quality tuna has been booming in recent years, for uses such as sashimi. Globally this demand is seeing significant increases, with 15 countries catching and producing at least 50,000 tons in 2020. Most wild-caught tuna is rapidly frozen on board fishing boats and then delivered to consumers. However, quality is greatly affected by conditions at the time of catch and handling during the distribution process. Conventional methods for inspecting the quality of frozen tuna usually require inspectors to cut off the fish's tail to visually examine a cross-section of the tuna tail after it is landed. This process limits the timing of these evaluations and relies on a limited number of skilled evaluators. The evaluation is also limited to the tail area.
Ultrasound waves are applied in quality testing in various fields as a non-destructive testing method. However, using them for frozen products such as tuna proved difficult due to the high attenuation of acoustic waves.
To address these issues, the joint research team examined frozen tuna with low-frequency, low-attenuation ultrasound waves to inspect the freshness of the fish. By analyzing the waveforms using machine learning, the two parties successfully developed the world's first method to determine the freshness of frozen tuna in a non-destructive manner.
A vital study area was determining the optimum frequency for examining frozen tuna. Tests showed that ultrasound waves with a relatively low frequency of about 500 kHz delivered optimum results. The study also aimed to develop an AI technology to identify insufficient freshness from ultrasound waves.
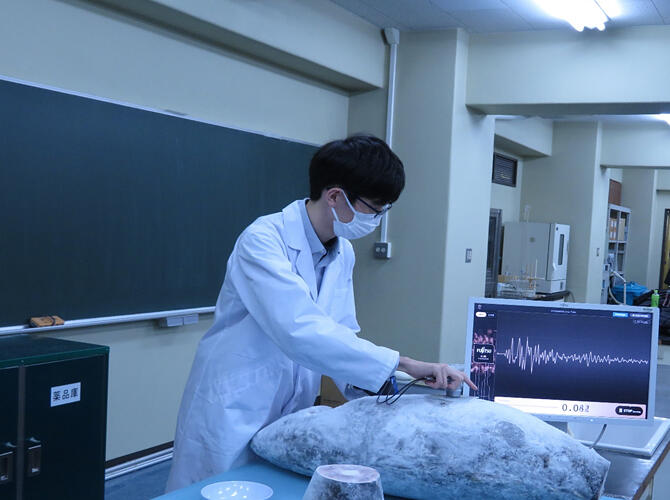
Provided by Tokai University
The group discovered that reflection intensity in the middle bone area of tuna specimens with insufficient freshness was especially intense. Based on these findings, it then created a machine learning model based on reflected waves from the middle bone of the tuna specimens that can non-destructively evaluate freshness.
Possible usage scenarios include wholesalers for marine products being able to easily inspect the freshness of a whole fish with a handheld device when buying tuna from fishermen. It could also be applied to inspections at fishing port facilities using belt conveyors for automated batch inspections of the freshness of frozen tuna.
Moving forward, the group will continue to increase the accuracy of this newly developed technology and further enhance it to detect other quality defects, including blood clots and tumors. The group also plans to conduct field trials at processing plants for marine products and conduct research to apply the technology to a wide range of areas, including the livestock industry, which handles frozen products, and the medical and biological fields.
This article has been translated by JST with permission from The Science News Ltd. (https://sci-news.co.jp/). Unauthorized reproduction of the article and photographs is prohibited.