A joint research group led by Visiting Researcher Jianshi Jin and Team Leader Katsuyuki Shiroguchi at the Laboratory for Prediction of Cell Systems Dynamics, RIKEN Center for Biosystems Dynamics Research, has succeeded in developing a fundamental technology for predicting cell phenotypes and gene expression states determined previously through comprehensive measurement using images and videos of single cells.
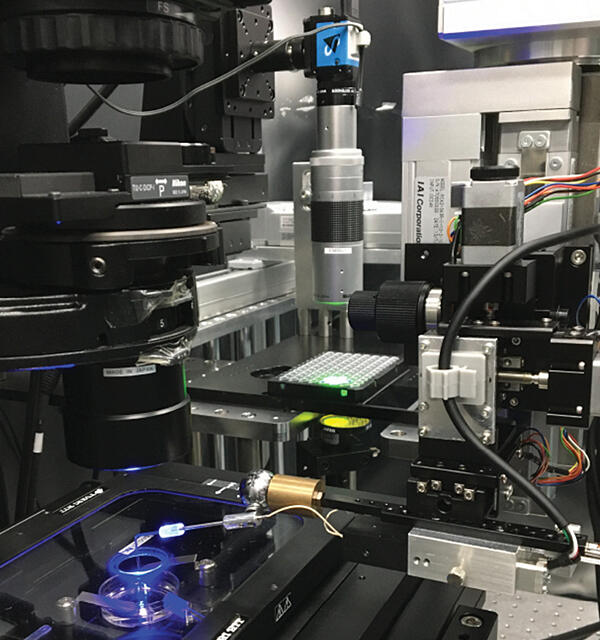
Provided by the RIKEN Center for Biosystems Dynamics Research
Shiroguchi said, "People can guess whether someone is well by looking at their complexion. We thought that if we could do this with cells, it would allow for non-invasive estimation of the internal state of cells from images."
To achieve this, the group developed an automated live imaging and cell-picking system, ALPS, which can automatically perform repeated single-cell fractionation and microscopic observations. ALPS automatically detects and picks individual cells regardless of their position on the stage. As a result, it can fractionate and isolate 96 cells in around 16 minutes.
A demonstration experiment using a population of cells from the blood circulatory system showed that it was possible to perform a data-driven determination of the gene expression of more than 1000 cells observed and isolated by ALPS using a next-generation sequencer (single-cell RNA sequencing method). This relationship between the cell imagery and gene expression states was extracted using AI analysis (deep learning), and the system successfully estimated the type and state of cells from the images and videos.
Shiroguchi said, "I believe that information science will develop to enable predictions of difficult-to-measure areas. Therefore, we want to develop methods to obtain data containing advanced information that will enable us to make these predictions. We want to improve the quality of the acquired images while expanding targets to include adhered cells, etc., enabling the state of various cells to be estimated with high precision. We would also like to integrate predictive technologies, such as the likelihood of a cell becoming sick or being infected with a virus, using comprehensive measurements to identify a cell's internal state."
Journal Information
Publication: Proceedings of the National Academy of Sciences of the United States of America
Title: Robotic data acquisition with deep learning enables cell image-based prediction of transcriptomic phenotypes
DOI: 10.1073/pnas.2210283120
This article has been translated by JST with permission from The Science News Ltd. (https://sci-news.co.jp/). Unauthorized reproduction of the article and photographs is prohibited.