It is common for chemists to intuitively predict better structures, including steric and electronic effects, from the results of examinations, and then actually synthesize and examine them in the pursuit of optimization in asymmetric catalysts. This has led to significant differences in catalyst design and performance, depending on the experience and intuition of the experimenter, even when different individuals have obtained the same experimental results. Methods have been developed to construct predictive models using machine learning and to quantitatively evaluate experimental results, but to make accurate predictions requires information on molecular structures and electronic states based on quantum chemical calculations, which require considerable computational resources and time.
A research group led by Specially Appointed Assistant Professor Nobuya Tsuji, Associate Professor Pavel Sidorov, Specially Appointed Associate Professor Yuuya Nagata, Principal Investigator Alexandre Varnek and Principal Investigator Benjamin List of the Institute for Chemical Reaction Design and Discovery at Hokkaido University, has successfully predicted and demonstrated the structure of highly selective chiral organic catalysts by machine learning using a newly developed molecular descriptor, without using quantum chemical calculations or other methods. The group's research was published online in Angewandte Chemie International Edition.
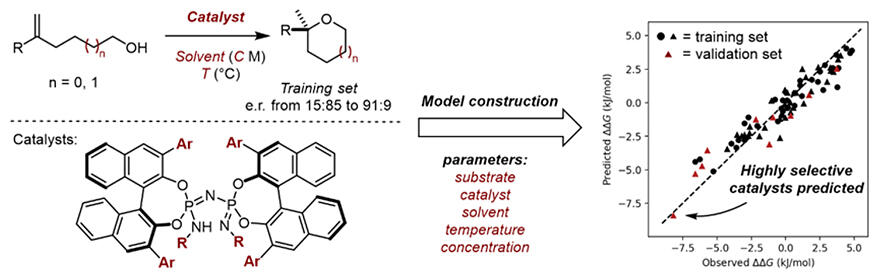
Provided by Hokkaido University
A new molecular descriptor, CircuS (Circular Substructures), has been developed in the framework of the ISIDA (In Silico Design and Data Analysis) descriptor developed by Varnek and colleagues. The descriptor was then applied to an asymmetric intramolecular cyclisation reaction using IDPi catalysts, an asymmetric organic catalyst developed by List and his colleagues, whereupon the molecular descriptor CircuS was evaluated. They combined the results of the experiments obtained through automated experiments using the automatic synthesis system to build a machine‐learning prediction model. The resulting predictive model was then used to evaluate unknown IDPi catalysts by virtual screening, and those predicted to have high performance were actually synthesized and examined.
The CircuS descriptor was developed in the hope that it would provide a more accurate representation of a molecule by providing branching and ring information for each resulting molecular fragment. Comparative studies have shown that the CircuS descriptor gives the best correlation compared to the commonly used fingerprint descriptors and ISIDA descriptors. In addition, by automating the process from experiment to analysis by means of automatic synthesis equipment, the researchers successfully developed a framework to seamlessly link the process from experiment to prediction with machine learning. The predictive model thus obtained was used to evaluate the selectivity given by unknown molecules and to rapidly identify the structure of a highly selective asymmetric organic catalyst.
The predictive model used in this method also includes parameters such as solvent and concentration, so that not only the structure of the catalyst but also the experimental conditions can be evaluated as well. As a result, this allowed the catalyst and reaction conditions that give the tetrahydropyran ring with high selectivity to be found. In addition, a program was developed to visualize the contribution of catalytic structures to selectivity in this approach, creating a framework that allows chemists to further design molecules from experimental results without relying on empirical subjectivity.
To assess the generality of the methodology, the researchers also applied it to the results of other response studies. Despite being completely different modes of reaction with different skeleton catalysts, the CircuS descriptor was found to give the best correlation compared to other 2D descriptors, indicating that the method is widely applicable and not limited to specific catalysts and reactions.
The experimental‐to‐prediction framework the group developed is expected to be used not only for asymmetric organic catalysis but also for a wider range of catalytic optimizations in general. It is expected to contribute to the further development of organic chemistry by accelerating the screening process in a more quantitative and reproducible manner compared to traditional optimization relying on intuition.
Journal Information
Publication: Angewandte Chemie International Edition
Title: Predicting Highly Enantioselective Catalysts Using Tunable Fragment Descriptors
DOI: 10.1002/anie.202218659
This article has been translated by JST with permission from The Science News Ltd. (https://sci-news.co.jp/). Unauthorized reproduction of the article and photographs is prohibited.