The identification of those who will benefit from treatment rather than those who are at high risk of developing the disease overall can yield significant therapeutic benefits. Therefore, those at high risk but in whom treatment is predicted to be less effective may be prescribed a different treatment option.
A research group led by Assistant Professor Kosuke Inoue of the Kyoto University Graduate School of Medicine (also a Program‐Specific Associate Professor at the HAKUBI Center for Advanced Research), Associate Professor Yusuke Tsugawa of the University of California, Los Angeles; Professor Susan Athey of Stanford University; and other colleagues developed a method to identify individuals in whom hypertension treatment using state‐of‐the‐art machine‐learning models would be highly effective. Inoue said, "In this study, we presented an approach to examine the effectiveness of treatment for hypertension in each patient, but the same concept can be applied to other treatments as well. In the future, we would like to link this to the development of programmed medical devices, and other relevant areas." The result was published in the International Journal of Epidemiology.
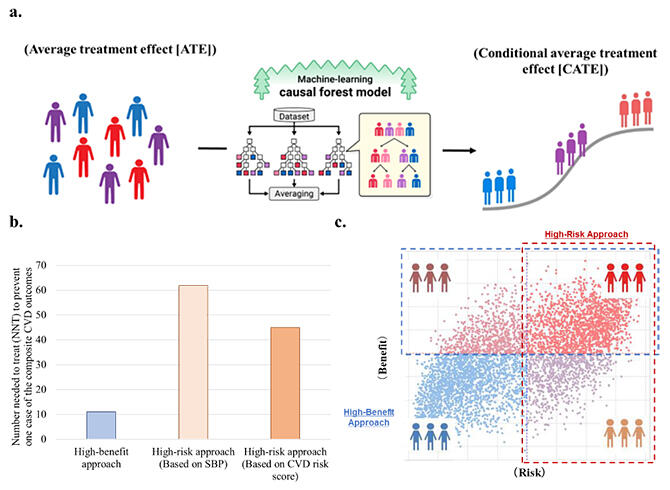
b. Selection of the treatment population by the high‐benefit approach showed an effect on the population about five times larger than the conventional high‐risk approach.
c. The individuals covered by the high‐benefit approach (blue box) and the high‐risk approach (red box) do not necessarily overlap.
Provided by Kyoto University
In the past, a high‐risk approach has been used, prioritizing the treatment of patients with cardiovascular diseases (CVD) and those at a high risk of mortality. In contrast, the research group proposed a high‐benefit approach that treats individuals predicted to benefit from treatment. However, to predict treatment efficacy, it is necessary to compare treatment effects according to a combination of multiple test values rather than individual specific test values.
The research group used a machine‐learning model developed by Professor Athey and colleagues in the field of economics to estimate antihypertensive treatment effects at the individual level. They applied causal forests (the machine learning algorithm used to estimate the treatment effects) to examine data from the ACCORD‐BP trial and SPRINT, a large randomized controlled trial of hypertension treatment.
The risk of developing CVD at 3 years was estimated using the causal forest for 10672 participants randomized to a systolic blood pressure (SBP) target of <120 mmHg (intensive antihypertensive treatment group) or <140 mmHg (standard antihypertensive treatment group). Around 20 variables were collected, including sex, age and various inspection values.
As a result, individual patients were categorized into high‐benefit and high‐risk, high‐risk but low‐benefit, low‐risk but high‐benefit, and low‐risk and low‐benefit groups. Comparison between the number of patients in the high‐risk and high‐benefit groups to prevent one case of CVD events showed that the high‐benefit approach included 11 patients, while the high‐risk approach included 61 patients, indicating that the treatment effect of the high‐benefit approach is about five times greater than that of the conventional high‐risk approach. Similar results were obtained in a study of 14,575 people of the civilian non‐institutionalized U.S. population included among the NHANES data.
Inoue continued, "In conventional medicine, therapeutic intervention is generally targeted at high‐risk populations. We believe that the results of this study clarify that more efficient therapeutic intervention can be achieved by focusing not only on risk but also on benefit and will contribute to the debate on how to distribute limited medical resources. The high‐benefit approach advocated in this study may also lead to the correction of health disparities. We hope that as more evidence is accumulated in the future this can be developed as a next‐generation personalized medicine strategy."
This work was supported by the Japan Agency for Medical Research and Development (AMED; JP22rea522107), the Japan Society for the Promotion of Science (21K20900 and 22K17392), the Japan Endocrine Society and the Next-Generation Global Human Resource Development Project (LINSIGHT) funded by MEXT.
This article has been translated by JST with permission from The Science News Ltd. (https://sci-news.co.jp/). Unauthorized reproduction of the article and photographs is prohibited.