A collaborative research group led by researcher Shunichi Kosugi at the Laboratory for Statistical and Translational Genetics, RIKEN Center for Integrative Medical Sciences (currently a researcher at the Genetic Research Division, Research Support Center, Shizuoka General Hospital) and Team Leader Chikashi Terao (head of the Clinical Research and Immunology Department, Shizuoka General Hospital) has developed a new method for detecting structural variations (SVs) in whole-genome sequencing data with high accuracy. The study has been published in Cell Genomics.
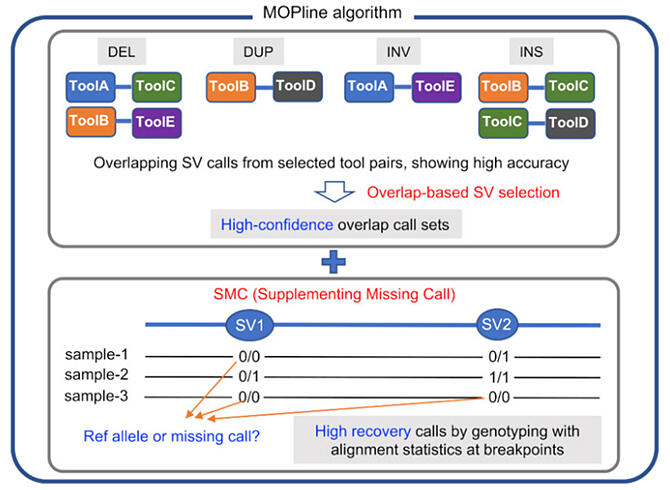
Provided by RIKEN
Generally, an SV in a genome refers to any deletion, insertion, duplication, or inversion variation of 50 base pairs (bp) or more. SV is distinct from indels (deletions and insertions of less than 50 bp) and single nucleotide variants (SNVs), which are single-base substitutions. Many studies have shown that SVs, which cause large differences between individual genomes, are genetic factors in a variety of human diseases and traits, including developmental and intellectual disabilities. The structural complexity and large size of SVs make them more difficult to detect compared with SNVs.
Genomic variants are typically detected by aligning short sequence (read) data of 100-150 bp to the standard human genome sequence (reference sequence). SNVs and indels fit within the read length. However, larger SVs do not fit within the reads and must be detected using indirect evidence from reads that align across SVs, resulting in reduced detection accuracy and sensitivity. Although many SV detection tools have been developed, none of the tools have been able to detect SVs with high accuracy and sensitivity due to the low commonality of detection results.
The collaborative research group discovered that the existing tools commonly used to detect overlapping SVs that are said to be capable of high accuracy detection, do not necessarily show high accuracy. Therefore, the group searched for the optimal combination of existing tools.
They developed an algorithm to determine the optimal combination of tools by SV type and size based on 4−9 existing tools. The algorithm was named Merging Overlap calls from selected Pairs of algorithms (MOP). Although MOP could be used to select SVs with high accuracy, some were still missed. Genomic regions where SVs were not detected by MOP were scanned to confirm the presence of SVs. To confirm this, a unique genotyping method based on read alignment information was used. This SV re-discrimination method was named Supplementing Missing Calls (SMC). Finally, an SV detection method that combined MOP, SMC, and filtering and annotation functions was successfully developed and named MOPline.
The accuracy and sensitivity of MOPline for SV detection were verified using whole-genome sequencing data. MOPline exceeded the accuracy and sensitivity of existing tools. Furthermore, a comparison with existing pies that combine multiple tools for SV detection was performed using 100 whole-genome sequencing data obtained from a public database (1000 Genomes Project). The results showed that, aside from having comparable SV detection accuracy, MOPline was superior to existing tools in terms of the number of true positive SVs (particularly insertions) detected (detection sensitivity).
MOPline was then used to detect SVs among the whole-genome sequencing data of 3258 individuals listed at BioBank Japan (BBJ). As a result, approximately 134,000 SVs were detected (∼16,000 per individual), which was 1.7−3.3 times higher than the number detected per individual in previous large-scale SV research projects. The BBJ whole-genome sequencing data were from patients with at least one disease, such as cancer or dementia.
Therefore, SVs overlapping the protein-coding regions of known disease-related genes were examined. Several rare SVs were found to overlap the protein-coding regions of known disease-specific risk genes (e.g., colorectal cancer, breast cancer).
Using the MOPline-detected BBJ SVs as a reference panel, imputation of single nucleotide polymorphism (SNP) array data (SNP genotype data) from 180,000 individuals was performed to analogize the SVs. Genome-wide association studies for 42 diseases and 60 quantitative traits were performed using the analogous SVs and medical information retrieved from approximately 180,000 individuals. The results revealed that 41 SVs correlated equally or more strongly with SNPs for 32 traits, including cancer and other diseases. Of the 41 correlated SVs, 8 overlapped the coding regions of related genes, and 5 of these were newly identified SVs with no previously reported association with the trait.
MOPline is a tool that demonstrates unprecedented SV detection accuracy and sensitivity, facilitating not only the identification of rare SVs that cause single-gene diseases but also of those involved in complex quantitative traits through SV imputation.
This article has been translated by JST with permission from The Science News Ltd. (https://sci-news.co.jp/). Unauthorized reproduction of the article and photographs is prohibited.