A joint research group composed of Graduate Student Kyoka Hara and Professor Noritaka Usami of the Graduate School of Engineering, Nagoya University; Researcher Takuto Kojima and Associate Professor Hiroaki Kudo of the Graduate School of Informatics, Nagoya University; and Researcher Kentaro Kutsukake at the RIKEN Center for Advanced Intelligence Project (AIP) successfully predicted the crystal orientation distribution of polycrystalline materials accurately using a machine learning model based on optical images. This technique enabled the easy and rapid structural analysis of various polycrystalline materials. The findings were published in APL Machine Learning.
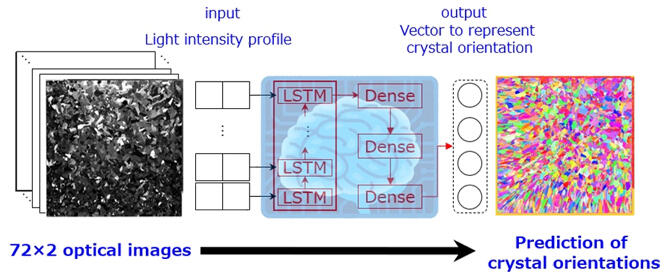
Provided by Nagoya University
Many common materials, such as metals, ceramics and semiconductors, are polycrystalline by nature. Polycrystals have complex structures composed of multiple crystal grains, and their properties vary remarkably based on the microscopic orientation distribution of these grains. For example, the strength and corrosion resistance of structural materials and the energy conversion efficiency of solar cells using polycrystalline materials as light-absorbing layers depend on the orientation of the crystal grains and the characteristics of grain boundaries, the interfaces between crystals. Therefore, controlling the crystal grain orientation distribution is crucial to obtain polycrystalline materials with excellent properties. Measurement of the crystal grain orientation distribution is of utmost importance in materials development.
Crystal grain orientation distributions are typically measured using electron backscatter diffraction (EBSD), which involves analyzing diffraction patterns while scanning with an electron beam using a scanning electron microscope. This technique exhibits excellent spatial resolution owing to the use of electron beams. However, it requires expensive equipment and long scan times to measure large-area samples. The same is true for X-ray measurements.
The joint research group undertook the challenge of predicting crystal orientations by applying a machine learning model to optical images taken from various directions after treating the surfaces of polycrystalline materials with a solution. The samples studied were polycrystalline silicon substrates measuring approximately 15 centimeters square; these substrates are used in practical solar cells.
First, anisotropic etching was performed by immersing the polycrystalline silicon substrates in an alkaline solution to create a surface condition where the reflectivity to visible light depends on the crystal grain orientation. Optical photographs were then taken by illuminating the surface with white light from various angles.
The crystal grain orientation distributions of multiple substrates were collected using a specialized X-ray device for over 100 hours and used as training data for a machine learning model. The machine learning model is a long short-term memory (LSTM) network that takes the light intensity profiles of each pixel in optical photographs as input and outputs a vector representing the crystal grain orientation. By applying data augmentation techniques such as rotation of the input light intensity profiles, the joint research group successfully achieved high accuracy with a median prediction error less than 3 degrees.
The total time required for measurement, including optical photography, machine learning model training, and orientation prediction, was approximately 1.5 hours, which was significantly faster than conventional techniques.
The findings of this study demonstrated the potential in principle for expanding the prediction of crystal grain orientation distributions in various polycrystalline materials. The developed technique can be considered an innovative technology for materials development.
Journal Information
Publication: APL Machine Learning
Title: A machine learning-based prediction of crystal orientations for multicrystalline materials
DOI: 10.1063/5.0138099
This article has been translated by JST with permission from The Science News Ltd. (https://sci-news.co.jp/). Unauthorized reproduction of the article and photographs is prohibited.