Project Associate Professor Takao Yoshikane and Professor Kei Yoshimura of the Institute of Industrial Science (IIS) at the University of Tokyo have developed a method using machine-learning for estimating high-resolution precipitation characteristics from low-resolution climate model simulations. Estimating detailed precipitation characteristics by region will be possible, which is difficult to do with current climate model simulations. The method has been published in Scientific Reports.
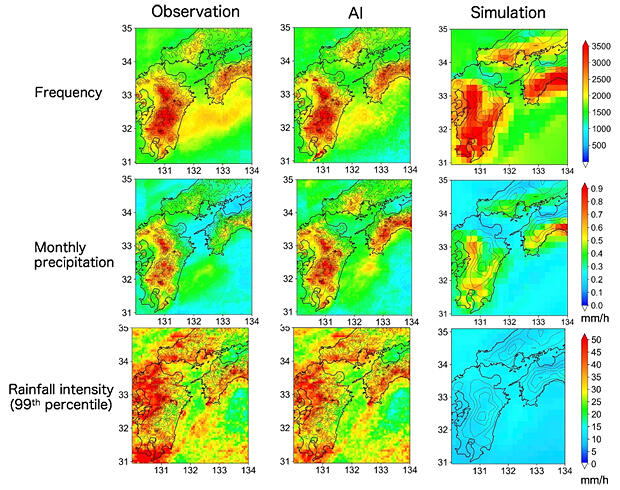
Provided by the University of Tokyo
To predict the risks of water-related disasters such as flooding, and climate change on the availability of water resources, the ability to reproduce detailed precipitation characteristics by region is essential.
Generally, a long-term climate model simulation is needed to reproduce climate change with a numerical model. However, because a huge amount of computer resources is required to perform such simulations at high resolution, current estimations of the climate change characteristics of local precipitation are difficult to do.
The research group developed a method to increase the resolution of low-resolution climate model simulations by applying a previously developed model bias correction method that uses machine learning to recognize patterns in the relationship between the spatial distribution characteristics of precipitation over a wide area and local precipitation.
Taking advantage of the ability of numerical models to reproduce meteorological phenomena at 5 to 8 times the resolution, higher resolution was achieved by applying discriminators to the climate model simulations. These discriminators are based on pattern recognition of the relationship between the spatial distribution characteristics of precipitation from forecast models and observed values.
The higher resolution was achieved by dividing the central grid of explanatory variables into nine parts, which increased the resolution from 0.18 degrees (∼ approximately 20 km) to 0.06 degrees (∼ around 5 km).
Additionally, by analyzing high-resolution precipitation estimates obtained from climate model simulations for 3,000 years, the research group clarified the climate change characteristics of precipitation during the rainy season in recent years.
Comparison with observed data revealed that the frequency and intensity of heavy rainfall over the past 60 years had been extremely influenced by natural variability rather than global warming. Furthermore, the high-resolution results obtained through AI and climate model simulation confirmed that the precipitation frequency and monthly precipitation in almost all areas had increased significantly.
By contrast, the number of sites with significantly increased heavy rainfall was approximately 35% of the total, which is related to the complex thermodynamic processes in mountainous regions.
These results indicate that the climatic characteristics of local precipitation (frequency, monthly amount, and heavy rainfall) can be reproduced with high resolution. In the future, it will be possible to apply the estimated values obtained with this method to a terrestrial water cycle simulation system. Moreover, the method can be used to discover vulnerabilities and strengthen countermeasures to reduce the risk of water-related disasters.
Journal Information
Publication: Scientific Reports
Title: A downscaling and bias correction method for climate model ensemble simulations of local-scale hourly precipitation
DOI: 10.1038/s41598-023-36489-3
This article has been translated by JST with permission from The Science News Ltd. (https://sci-news.co.jp/). Unauthorized reproduction of the article and photographs is prohibited.