A research group led by Graduate Student Takuma Sumi, Associate Professor Hideaki Yamamoto and Professor Ayumi Hirano-Iwata of the Research Institute of Electrical Communication (RIEC) at Tohoku University, and Professor Yuichi Katori of the School of Systems Information Science at Future University Hakodate has announced that they have analyzed the computational capabilities of an 'artificially cultured brain' constructed from rat cerebrocortical neurons based on reservoir computing, which is a machine learning paradigm, and have observed that it improves the processing of time series data.
An experimental system was constructed to analyze the computational capability of the 'artificially cultured brain.' They have also shown that the computer is capable of category learning and can classify the speech of a male speaker using an output layer trained on a female speaker, which is difficult to accomplish with ordinary machine learning. This achievement is expected to lead to the development of machine learning models and hardware that mimic the neural circuits of living organisms more closely and was published in the June 12, 2023 issue of the Proceedings of the National Academy of Sciences 'PNAS.'
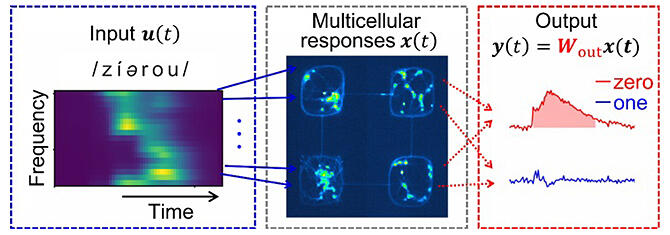
©Yamamoto et al.
The biological brain has been used as a model to achieve high information processing performance and low power consumption in devices, leading to many advances. By contrast, recent AI technologies require huge amounts of power and data capacity for training. For example, 1000 MWh is needed to learn the GPT-3 language model, while the human brain uses a total metabolic energy of approximately 3.5 MWh for efficient learning by the age of 20. This is expected to be overcome by acquiring new knowledge about biological information processing and its mimicry.
So far, the research group has established a technique for constructing an 'artificially cultured brain' by culturing nerve cells taken from rat brains on a special glass substrate, to clarify the principle behind the working of the brain's neural circuits.
In this research, they analyzed the computational capability of the 'artificially cultured brain' using reservoir computing.
Reservoir computing is a model for recurrent neural networks used in machine learning to improve learning efficiency and reduce computational cost and was originally proposed to model information processing in the mammalian cerebral cortex.
The 'artificially cultured brain' used in this experiment was formed by transfecting light-sensitive ion channel into neurons from rat cerebral cortex and then by culturing these neurons on a special glass substrate. On the special glass substrate, four 200-µm square areas (modules) were connected with thin lines in the middle of adjacent sides, and the neurons were grown on these modules and on the lines. The cells were designed to grow on the modules and on the line. The input signal was a 0 (zero) or 1 (one) audio.
Sound was input to the cells via Fourier transformation as a light stimulus, and the cells that receive the light were activated. There were approximately 20 cells in one module, and one frequency band was assigned to each cell.
The experimental results showed that the correct response rate for the two-class classification task (zero/one) was 82.5 ± 21.9%, demonstrating that the neural network generated by living cells has a short-term memory of a few hundred milliseconds, which can be used to classify speech utterances. The research group also found that neural circuits with strong modularity have consistently high correct response rates.
The results also showed that the output layer trained on female speakers can be used to classify the speech of male speakers. The 'artificially cultured brain' was shown to function as a generalization filter to improve the computational performance of reservoir computing. In the future, the research group expects that these findings will open up the possibility of wetware realization in addition to hardware and software.
Journal Information
Publication: PNAS
Title: Biological neurons act as generalization filters in reservoir computing
DOI: 10.1073/pnas.2217008120
This article has been translated by JST with permission from The Science News Ltd. (https://sci-news.co.jp/). Unauthorized reproduction of the article and photographs is prohibited.