Traffic congestion in Japan not only induces stress but also results in annual losses of approximately 10 trillion yen and contributes to greenhouse gas emissions. Assistant Professor Koh Takeuchi and Professor Hisashi Kashima of Kyoto University's Graduate School of Informatics, and the Sumitomo Electric System Solutions Group have developed a new spatiotemporal artificial intelligence (AI) technology, queueing-theory-based neural network (QTNN), to predict the location and length of upcoming traffic jams. In experiments predicting queue length one hour ahead on Tokyo roads, the company achieved highly accurate predictions with an average error of less than 40 m. The results were presented at the 29th ACM SIGKDD Conference on Knowledge Discovery and Data Mining, an international conference on machine learning and data mining.
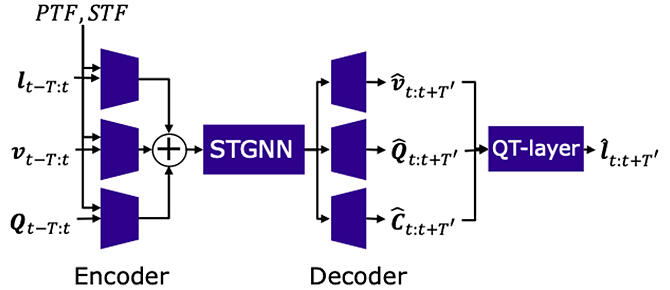
Provided by Kyoto University
Given that traffic congestion can be predicted, traffic flow can be smoothed using anticipatory route guidance and signal control, allowing for the prevention of traffic jams. However, traffic congestion is highly variable in terms of time of day, location of occurrence, and length, and its occurrence rapidly changes traffic conditions. Developing an AI that can accurately predict its presence or absence and length has been a formidable challenge. Furthermore, the practical application of traffic jam forecasting AI requires building AI interpretability and reliability, for example, clarifying the reasons for traffic jam forecasts.
In response to this issue, the research group developed a new highly accurate and interpretable spatiotemporal AI technology by combining traffic engineering knowledge based on years of field experience, deep learning, and traffic big data.
The developed spatiotemporal AI technology, QTNN, is based on traffic engineering theory and can learn the relationship between congestion changes and the road network via big data. QTNN first uses deep learning to predict the average speed and traffic volume at each intersection on the basis of congestion and road network data for numerous roads. Next, it predicts the traffic congestion length while correcting the traffic flow model used in traffic engineering. Through this two-step prediction method, the group achieved traffic congestion prediction consistent with the knowledge from traffic engineering by using cutting-edge deep learning.
An experiment was performed using the data provided by the Tokyo Metropolitan Police Department to predict−−one hour in advance−− the length of traffic congestion on 1,098 roads in Tokyo over two months. The company achieved a highly accurate prediction with an average error of less than 40 m by accurately predicting instances of severe traffic congestion as well as no traffic congestion. The results indicate a 12.6% reduction in prediction error as compared to currently considered state-of-the-art deep learning methods. Furthermore, because QTNN uses a traffic flow model that represents the relationship between traffic volume, average speed, and congestion length, it was able to find that the number of incoming vehicles increases dramatically around 6:00 a.m., and the length of the congestion increases significantly. Traffic volumes and average speeds decrease as congestion peaks. However, owing to a certain amount of traffic on the surrounding roads, this congestion will continue until 10:00 a.m. Some interpretation of the forecast results is now feasible.
QTNN is being considered by the Metropolitan Police Department in its project to upgrade traffic control systems using AI and big data. They plan to conduct evaluation tests on some roads to verify the reliability of the technology in preparation for full-scale operation in a real-world environment. Furthermore, the project aims to realize a spatiotemporal AI technology that will serve as a foundation for cities, flexibly utilizing information on signal control, road construction, and accident occurrence to predict traffic jam length.
Takeuchi commented, "In the fierce AI research competition that is taking place around the world, we believe that Japan's expertise in detailed data measurement technology and in-depth domain knowledge, combined with cutting-edge spatiotemporal AI technology, will give us a significant advantage over the rest of the world. This research aims to offer new solutions for traffic congestion and significantly contribute to urban sustainability. We will continue to pursue the potential of reliable and secure AI technology."
This article has been translated by JST with permission from The Science News Ltd. (https://sci-news.co.jp/). Unauthorized reproduction of the article and photographs is prohibited.