A joint research team led by Director Masahiko Demura, Deputy Platform Director Satoshi Minamoto, and their colleagues of the Research Network and Facility Services Division at the National Institute for Materials Science (NIMS), together with Professor Toshiyuki Koyama of Nagoya University, has devised a new two-stage heat treatment method that enhances the high-temperature strength of nickel−aluminum alloys beyond the capability of conventional methods. To achieve this, the materials researchers analyzed excellent heat treatment patterns discovered by AI, obtaining insights that contribute to the material science field. This is a good example of how collaboration between AI and materials researchers has created a new way of thinking. The results were published in Scientific Reports.
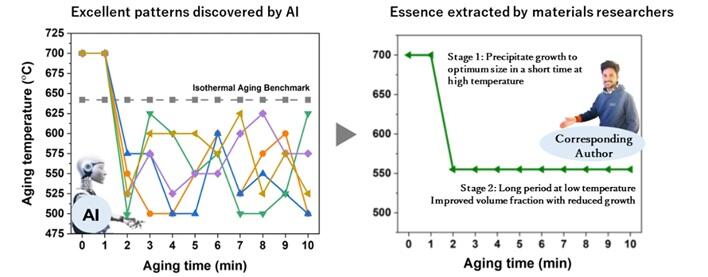
Provided by NIMS
Nickel−aluminum alloys are composed of a gamma phase, in which nickel and aluminum are randomly arranged, and a gamma prime phase (precipitated phase), in which nickel and aluminum are regularly arranged. To improve the high-temperature strength of this alloy, the size and volume fraction of the gamma prime phase must be controlled at appropriate values. The size and volume fraction of the gamma prime phase are determined based on heat treatment conditions. However, the number of heat treatment patterns consisting of combinations of temperature and holding time is extraordinarily large. For example, if the total time is divided into 10 equal parts and the temperature is set to 9 levels, the number of heat treatment patterns is approximately 3.5 billion (9 to the 10th power).
The research team used an AI algorithm called Monte Carlo Tree Search to efficiently search for the best pattern among a large number of combinations. The heat treatment patterns diverge into different paths as time progresses, each leading to different outcomes. Therefore, the research team utilized the algothrim, a technique commonly used in board games such as Shogi and Igo, to explore promising moves.
They conducted 1620 iterations of trial and error, which included calculating the high-temperature strength for patterns suggested by AI through numerical calculations and then feeding back the results to the AI for use in the next proposal. As a result, they discovered 110 patterns that surpass conventional heat treatment patterns. The discovered patterns were complex combinations of heating and cooling, which humans would not have thought of.
When experts analyzed why the patterns discovered by AI were superior, they found common features in these outstanding patterns. First, the gamma prime phase was rapidly grown at high temperatures and then cooled down before the optimal size (approximately 41 nm) was exceeded. Conditions were set to prevent further growth beyond this size. Subsequently, by maintaining a low-temperature range for an extended period, efforts were made to restrain growth as much as possible, thereby ensuring that the size did not exceed the optimal value. During this process, the volume fraction was slowly increased, thereby enhancing the high-temperature strength. The gamma prime phase grows faster at higher temperatures, where atoms diffuse faster. Thermodynamically, the gamma prime phase is more stable at lower temperatures, and its volume fraction increases as the temperature is decreased. The patterns discovered by AI effectively utilize these features without prior training.
From the patterns discovered by AI, the research team realized that the key point to improve high-temperature strength lies in combining high-temperature short-duration treatment with low-temperature long-duration treatment. Furthermore, they found that it is not necessary to repetitively raise and lower the temperature in small increments. Therefore, they devised a two-stage heat treatment method that combines two isothermal treatments: high-temperature short-duration and low-temperature long-duration treatments. Based on this idea, by optimizing the temperature during the second stage (low-temperature long-duration treatment), they were able to design a heat treatment pattern that surpasses the best pattern discovered by AI.
The devised two-stage heat treatment is based on a novel concept that had never been considered before, and it has been conceptualized for the first time through the analysis of AI discoveries by experts. In games like Shogi and Igo, new strategies have emerged from professional players deeply analyzing AI's game records. Similarly, this study has demonstrated that new perspectives in the field of materials can emerge through collaborative work between AI and experts.
Nickel−aluminum alloys are model alloys for nickel-based superalloys, which are heat-resistant materials. In the future, the research team aims to find ways to improve the process that can improve the fuel efficiency of gas turbines by applying the alloy to more practical alloy systems. This will eventually contribute to the realization of a low-carbon society.
Journal Information
Publication: Scientific Reports
Title: Artificial intelligence inspired design of non-isothermal aging for γ−γ′ two-phase, Ni−Al alloys
DOI: 10.1038/s41598-023-39589-2
This article has been translated by JST with permission from The Science News Ltd. (https://sci-news.co.jp/). Unauthorized reproduction of the article and photographs is prohibited.