It is widely recognized that the accuracy of AI-based predictions depends on the volume of training data. However, Professor Toshiaki Taniike and his colleagues from the Materials Chemistry Frontier Research Area at the Japan Advanced Institute of Science and Technology (JAIST), in collaboration with Professor Keisuke Takahashi from the Faculty of Science at Hokkaido University, have developed an automatic feature engineering technique. This technique facilitates highly accurate predictions of material functions using machine learning, even without extensive prior knowledge of the subjects. The study was published in the online edition of Communications Chemistry.
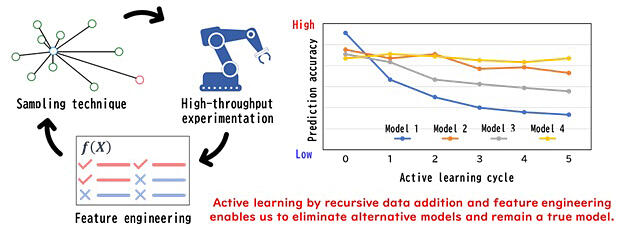
Provided by the Japan Advanced Institute of Science and Technology (JAIST)
Machine learning, including AI, is increasingly used to expedite research and development in practical materials, such as catalysts. This process involves utilizing data to train machines and variables (descriptors) to describe the materials and predict their functions. In particular, for accurately predicting the functions of unknown materials, it is crucial to have material descriptors that efficiently and comprehensively capture factors influencing the functions.
Traditionally, these descriptors were designed based on the profound domain knowledge of individual researchers on the subjects. Machine learning has not been fully applicable in areas with insufficient accumulated knowledge.
The research group developed a general-purpose automatic feature engineering (AFE) technique that enables design of effective descriptors without requiring any prior knowledge about the material of interest. Tailored for small datasets of materials, the AFE technique generates a large number of candidate descriptors from general physicochemical properties relating to elements and like factors through mathematical operations, allowing the machine to select descriptors relevant to the target materials. If each descriptor candidate is considered as a "hypothesis" that can explain the function of a material, then the AFE technique can be regarded as a hypothesis screening method that generates numerous hypotheses on the computer and selects the important ones.
For distinct targets, including the oxidative coupling of methane, the conversion of ethanol to butadiene, and light-off temperatures in three-way catalysis, the AFE technique demonstrated far superior prediction accuracy over traditional methods, which rely on elemental compositions of catalysts as descriptors. Moreover, when combined with high-throughput experimentation, this approach enabled the machine to accurately recognize catalyst designs, proving its versatility. It finally succeeded in pinpoint prediction of, at an accuracy rate of 83%, various high-performance catalysts with a low similarity level.
The success of this method was underpinned by the high-throughput experimental technique developed by Taniike's group and the high-quality catalyst data it generated.
The AFE technique is highly versatile, and its applications extend beyond catalysts. It can be readily used for various material targets by simply changing the training datasets. The research group highlighted that, by integrating the AFE technique with high-throughput experiments and farthest point sampling, it becomes feasible to efficiently discover candidate materials from an immensely vast feature space, potentially containing billions of different materials, without needing any prior knowledge or hypotheses. As a next step, the AFE technique will be made available as software, facilitating its widespread implementation in society.
Journal Information
Publication: Communications Chemistry
Title: Automatic feature engineering for catalyst design using small data without prior knowledge of target catalysis
DOI: 10.1038/s42004-023-01086-y
This article has been translated by JST with permission from The Science News Ltd. (https://sci-news.co.jp/). Unauthorized reproduction of the article and photographs is prohibited.