A joint team, led by Professor Dam Hieu Chi of the Co-Creative Intelligence Research Area at the Japan Advanced Institute of Science and Technology (JAIST), has announced the development of a new materials informatics method using deep learning with an attention mechanism. When applied to molecular and crystalline materials, the method demonstrates high accuracy in predicting the physical properties of a wide range of materials, while concurrently allowing explicit interpretation of structural properties. The results of the study were published in the December 7 issue of the scientific journal npj Computational Materials.
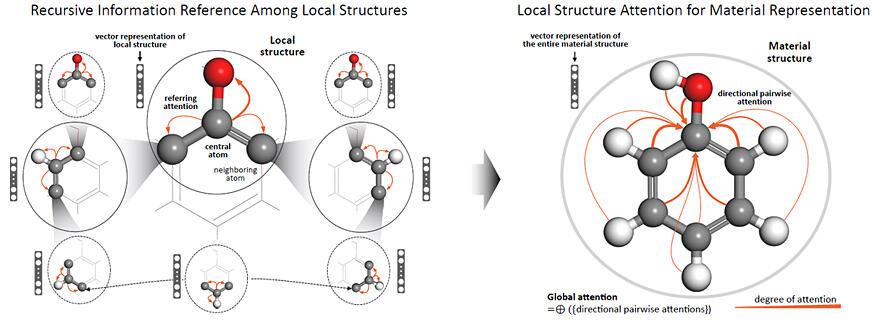
Provided by the Japan Advanced Institute of Science and Technology (JAIST)
In this study, the research team developed a method to quantitatively evaluate the degree of attention given to each local structure when predicting the physical properties of molecules and crystals. This was achieved by appropriately dividing the entire molecular or crystal structure into local structures and employing a deep learning model with an attention mechanism. The attention mechanism is an advanced technique in the field of deep learning, characterized by automatic attention to important information in the data.
The method recursively examines each local structure within the structure to gather information about one another, learning from the data in a unified and consistent manner. This allows for not only prediction of the physical properties of materials with a very high degree of accuracy but also identification of the reasons why these properties occur.
In addition, by quantitatively showing the degree of attention paid to each local structure in the structure of a material, it is possible to intuitively understand the complex relationship between physical properties and structure, reduce computational costs, and gain a deeper understanding of new materials, thereby accelerating material development.
The use of attention mechanisms in materials science is closely related to recent generative models that use self-attention mechanisms in image processing and natural language processing, such as OpenAI's GPT series and DALL-E, which are used for sentence generation. The mechanism automatically captures the inherent relationships and patterns in the data and is particularly effective at using them to generate new data.
Dam stated, "The true innovation of our research is not just the reduction in computational time and cost, but the deep insights and new hints of discovery about materials that it provides to researchers. It presents a groundbreaking approach to opening a new era in knowledge co-creation through human-AI collaboration. This has the potential to accelerate the discovery and development of new materials in science and industry and significantly change the nature of future scientific research."
Journal Information
Publication: npj Computational Materials
Title: Towards understanding structure-property relations in materials with interpretable deep learning
DOI: 10.1038/s41524-023-01163-9
This article has been translated by JST with permission from The Science News Ltd. (https://sci-news.co.jp/). Unauthorized reproduction of the article and photographs is prohibited.