A research team led by Professor Hiroyasu Ando (Advanced Institute for Materials Research, Tohoku University) and Professor Yuichi Katori (School of Systems Information Science, Future University Hakodate) has proposed the concept of computation harvesting, which utilizes nature itself as computational resources to predict the complex dynamics of natural phenomena. They successfully conducted a series of experiments to verify this concept. The results were published in the academic journal PLOS ONE.
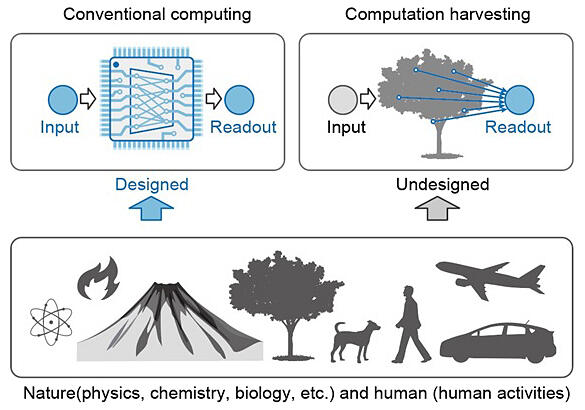
©Hiroyasu Ando, Yuichi Katori et al. Provided by Tohoku University
The research team set up an experimental system consisting of a camera to capture wind-exposed plants and their movements. In the experiment, they applied the principles of reservoir computing to the movement of detected feature points in the plant images, estimating wind direction and strength through a methodology that does not require detailed designs.
The results showed that it is possible to classify different wind directions and strengths from only a simple linear sum (addition of vectors) of the information extracted from plant images. Specifically, the method's robustness was confirmed by restricting the feature points utilized for classification. The results demonstrated that with appropriate selection, both wind direction and strength could be classified by performing additions for movements of only three feature points.
The research team has also demonstrated that even when analyzing an image where a plant is exposed to a wind pattern not included in the learning process, the wind can still be classified based on parameters learned from a different pattern. This suggests that there is a generalizability in the movement and structure of plants.
In the methodology of computation harvesting, images of existing surveillance cameras, which are used as Internet of Things (IoT) sensors, minimizes infrastructure costs and enables low-power computation. Furthermore, it achieves a computing system that utilizes the target as-is, eliminating the need for detailed design. Future research is expected to lead to the development of technologies that can predict wind speed and sunlight intensity from information sources such as video images of the environment, accessible via existing infrastructure. Furthermore, these developments will facilitate the predicting of renewable energy generation levels based on such environmental data.
Journal Information
Publication: PLOS ONE
Title: Computation harvesting from nature dynamics for predicting wind speed and direction
Takumi Aita, Hiroyasu Ando, Yuichi Katori
DOI: 10.1371/journal.pone.0295649
This article has been translated by JST with permission from The Science News Ltd. (https://sci-news.co.jp/). Unauthorized reproduction of the article and photographs is prohibited.