A research group led by Lecturer Shigeki Yamada at Nagoya City University Graduate School of Medical Science, in collaboration with Shiga University of Medical Science, the University of Tokyo, Osaka University, Tokyo Metropolitan University, Tohoku University, Yamagata University, and Fujifilm, has announced that they have developed an AI tool to extract disproportionately enlarged subarachnoid-space hydrocephalus (DESH), which is crucial for the diagnosis of Hakim disease (idiopathic normal pressure hydrocephalus: iNPH), from three-dimensional (3D) MRI brain images and provide a basis from extracted regions. The results are expected to contribute to the improvement of precision of iNPH diagnosis and were published in the March 15 issue of the international academic journal Frontiers in Aging Neuroscience.
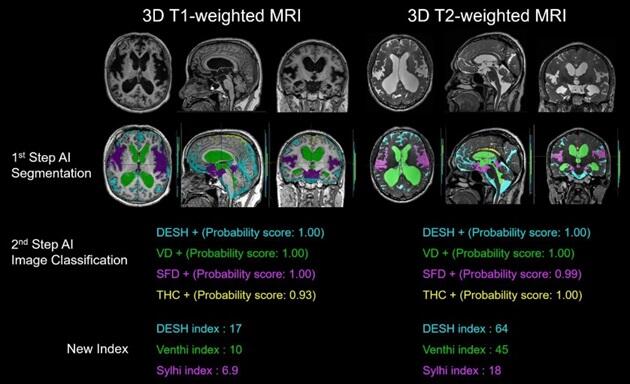
Provided by Nagoya City University Hospital
Hakim disease is a form of hydrocephalus in which cerebrospinal fluid builds up chronically in the skull, and its incidence rate increases with age. This condition presents with a triad of symptoms: gait disturbance, memory loss, and urinary incontinence. The disease is progressive, and once symptoms are aggravated, patients are prevented from performing daily activities and require nursing care. Moreover, recovery from the advanced stage of the disease is difficult. This means that early detection and treatment are considered important. As both ventricles and the subarachnoid space surrounding the brain enlarge simultaneously, Hakim disease is often mistaken for brain atrophy, resulting in delayed detection.
As an important imaging finding to discriminate Hakim disease from brain atrophy, Japanese researchers have previously reported the simultaneous occurrence of THC (tightened sulci in the high convexities) and SFD (Sylvian fissure dilatation) in Hakim disease and named it DESH. Although the diagnostic rate improved, determination of DESH was challenging. Determination is based on a subjective evaluation, meaning that even an expert judgment can be different from another.
In this study, the research group first developed an AI tool that automatically extracts the four intracranial regions necessary to evaluate DESH (cerebrospinal fluid space, ventricles, sulci in the high convexities and midline, and Sylvian fissure and basal cistern) from 3D MRI images. Specifically, three-dimensional T1-weighted and T2-weighted MRI data of 138 healthy volunteers aged 21−92 years and 43 patients diagnosed with Hakim disease were acquired using a 3-Tesla MRI system. Fujifilm's cloud-based AI technology development support service SYNAPSE Creative Space was used to efficiently extract the ventricles and subarachnoid space from each image. Moreover, two regions, THC and SFD, were manually extracted from the subarachnoid space. Three-dimensional T1-weighted MRI (from 160 participants) and T2-weighted MRI (from 180 participants) data of these four regions were prepared for deep learning. Deep learning of region extraction through semantic segmentation using 3DU-Net was performed until the accuracy sufficient for region extraction was reached.
Next, a convolutional neural network (CNN) was used for deep learning to determine the presence or absence of DESH from the cerebrospinal fluid space, ventricular enlargement from the ventricles, THC from the sulci in the high convexities and midline, and SFD from the Sylvian fissure and basal cistern through image classification. An AI tool that automatically makes the aforementioned determinations was developed. Furthermore, the DESH index, Venthi index, and Sylihi index were proposed as three indexes to quantify these judgments. They also confirmed that the degree and characteristics of DESH can be quantified.
Yamada said, "With the aim of discriminating between Hakim disease and brain atrophy seen in Alzheimer's disease, we developed an AI tool that automatically extracts the three regions necessary to determine DESH from MRI images, namely, the ventricles, the sulci in the high convexities and midline, and the Sylvian fissure and basal cistern. Subsequently, we obtained medical device approval as a "cerebrospinal fluid space analysis" application for SYNAPSE VINCENT, a three-dimensional workstation sold by Fujifilm that is powered by this AI and released it at the same time as the publication of the paper. The system is now available for use by clinicians. Even I, as an experienced person, need 2−3 hours per patient to extract these three regions from an MRI image, but this application takes only 2−3 seconds. Furthermore, while many two-dimensional indexes have been devised previously to objectively detect DESH, AI can now instantly measure highly accurate three-dimensional indexes that are distinct from conventional two-dimensional indexes, using the volume of the regions extracted automatically by this application."
Journal Information
Publication: Frontiers in Aging Neuroscience
Title: Automatic assessment of disproportionately enlarged subarachnoid-space hydrocephalus from 3D MRI using two deep learning models
DOI: 10.3389/fnagi.2024.1362637
This article has been translated by JST with permission from The Science News Ltd. (https://sci-news.co.jp/). Unauthorized reproduction of the article and photographs is prohibited.