Postdoctoral Researcher Miao Wang and Team Leader Ken Sakaushi of the NIMS Research Center for Energy and Environmental Materials and Researcher Akimitsu Ishii of the NIMS International Center for Young Scientists developed a method that can accurately predict electrocatalyst degradation for water electrolysis systems in a short experimental time. By combining data assimilation, which is also used for weather forecasting, the team succeeded in accurately predicting the degradation of a material that degrades rapidly in approximately 900 hours based on the results of a 300-hour experiment. Faster prediction of catalyst degradation results in effortless comparison of the degradation level of various catalytic materials. Such is expected to advance the understanding of the degradation mechanism and accelerate the development of highly efficient, inexpensive, and long-life catalytic materials. The results were published in ACS Energy Letters.
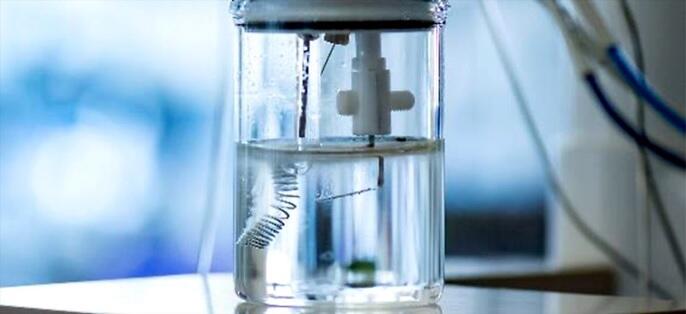
Provided by NIMS
The oxygen evolution reaction (OER) is an important counter-reaction in numerous electrochemical conversion processes, such as hydrogen evolution for green hydrogen production, carbon dioxide reduction for synthesizing high value-added molecules, and nitrogen reduction for ammonia synthesis. The durability of electrocatalysts is extremely important for the maintenance of energy efficiency so that OER electrocatalyst materials maintain high energy efficiency during the electrolyzer operation period of over 10 years. However, evaluating the durability of electrocatalysts requires a long period of operation, sometimes >10 years. Therefore, a method that can accurately predict the lifetime of electrocatalysts in a short period is essential for accelerating the development of advanced electrolyzers.
An effective data-driven method for such prediction is data assimilation (DA), which dynamically combines a mathematically defined simulation model with experimental data and dynamically modifies the parameters of the model as needed to obtain forecasted data in a short time with high accuracy. It is used in meteorology and oceanography for highly accurate weather forecasting.
The research team successfully developed a new kinetic electrocatalyst degradation model focusing on electrocatalyst dissolution in OER. To mathematically describe the degradation mechanism, they employed an energy span model, a multistep reaction theory, where free energy and potential are related by a first-order approximation, to describe the microscopic kinetic picture of OER simply. This approximate treatment allowed for quantitatively describing the relationship among applied potential, current, and time during the degradation process using a minimal number of parameters (two for the OER reaction and two more for electrocatalyst dissolution). By applying DA to this degradation model, the parameters in the model were efficiently optimized, and a DA-type degradation analysis approach was developed to predict the lifetime by extrapolating the potential-time relationship obtained from the model.
The developed DA-type degradation analysis approach achieved reliable degradation lifetime prediction with an error of <10% at 34% of the total experimental period. Meanwhile, other methods, such as simple fitting, failed to demonstrate the same predictive ability as the DA-type degradation analysis approach. They attributed this result to the fact that the proposed DA approach effectively integrated useful information contained in the experimental data and prior knowledge regarding degradation into the physicochemical model.
Journal Information
Publication: ACS Energy Letters
Title: Accelerated Electrocatalyst Degradation Testing by Accurate and Robust Forecasting of Multidimensional Kinetic Model with Bayesian Data AssimilationClic
DOI: 10.1021/acsenergylett.4c02868
This article has been translated by JST with permission from The Science News Ltd. (https://sci-news.co.jp/). Unauthorized reproduction of the article and photographs is prohibited.