Graduate Student Shusuke Ito, Assistant Professor Koki Muraoka, and Professor Akira Nakayama of the Department of Chemical System Engineering, Graduate School of Engineering at the University of Tokyo have developed an organic molecular design methodology using large language models (LLMs). Their research, published in Chemistry of Materials, demonstrates how LLMs can be utilized for organic molecular design by leveraging accumulated scientific knowledge and simulation results through natural language.
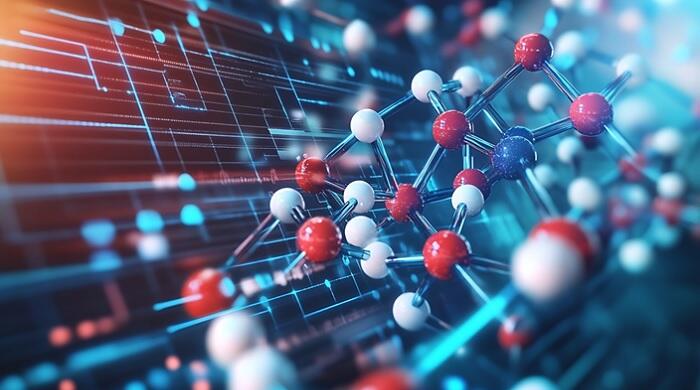
Designing organic molecules tailored to particular applications is a complex task requiring trial and error by experienced experimental chemists. As a result, even in the modern era where large-scale information processing via computers is possible, experimental chemistry still leads the development of new organic molecules.
To address this challenge, the researchers utilized LLMs to achieve collaborative organic molecular design combining experimental chemistry expertise with computational information processing capabilities. LLMs are machine learning models trained on vast amounts of text data that can generate human-like text covering topics across various fields.
As a case study, the team attempted to design organic structure-directing agents (OSDAs) that promote the crystallization of zeolite, an important inorganic porous material in the chemical industry. OSDAs typically adopt quaternary ammonium cation structures and facilitate the formation of specific crystal phases by fitting into spaces within the zeolite crystal structure. While OSDAs are widely used in industrial zeolite production, they account for a significant portion of manufacturing costs, making the search for lower-cost alternative molecules a major challenge. By applying their LLM-based system, the researchers successfully designed organic molecules that specifically fit the internal shapes of zeolites.
This approach enables the generation of experimentally validated organic molecules, organic molecules that are structurally similar to these molecules, and completely novel promising candidates. The methodology holds potential not only for molecular design but also for drug development and design of other complex compounds.
Journal Information
Publication: Chemistry of Materials
Title: Knowledge-Informed Molecular Design for Zeolite Synthesis Using General-Purpose Pretrained Large Language Models Toward Human-Machine Collaboration
DOI: 10.1021/acs.chemmater.4c02726
This article has been translated by JST with permission from The Science News Ltd. (https://sci-news.co.jp/). Unauthorized reproduction of the article and photographs is prohibited.